From Theory to Practice: Practical Data Mining Techniques for Students
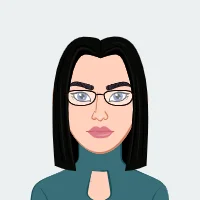
Embarking on the journey into data mining, students are met with a blend of excitement and challenges. While theoretical knowledge sets the stage, the true mastery of data mining techniques hinges on practical application. This blog is a compass, navigating the terrain of fundamental data mining methods to weave a seamless bridge between theory and practice. If you need help with your data mining homework, understanding fundamental data mining methods is crucial for applying theoretical knowledge to practical scenarios and achieving success in your academic endeavors.
In the realm of data mining, the journey begins with understanding theoretical foundations. Concepts, algorithms, and methodologies form the backbone, providing the scaffolding upon which practical applications are built. Theoretical knowledge acts as a compass, guiding students through the intricate landscape of data mining. It lays the groundwork for what comes next, a crucial understanding that transforms information into actionable insights.
However, the journey doesn't end with theory alone. Practical application is the linchpin for students aspiring to master data mining techniques. Classification and regression analysis emerge as fundamental tools, empowering students to predict and categorize data. Delving into clustering techniques, they discover the art of grouping similar data points, unraveling hidden patterns with every iteration. Association rule mining becomes a beacon, illuminating the pathways to uncover relationships within datasets. Time series analysis adds a temporal dimension, extracting meaningful information from chronologically ordered data.
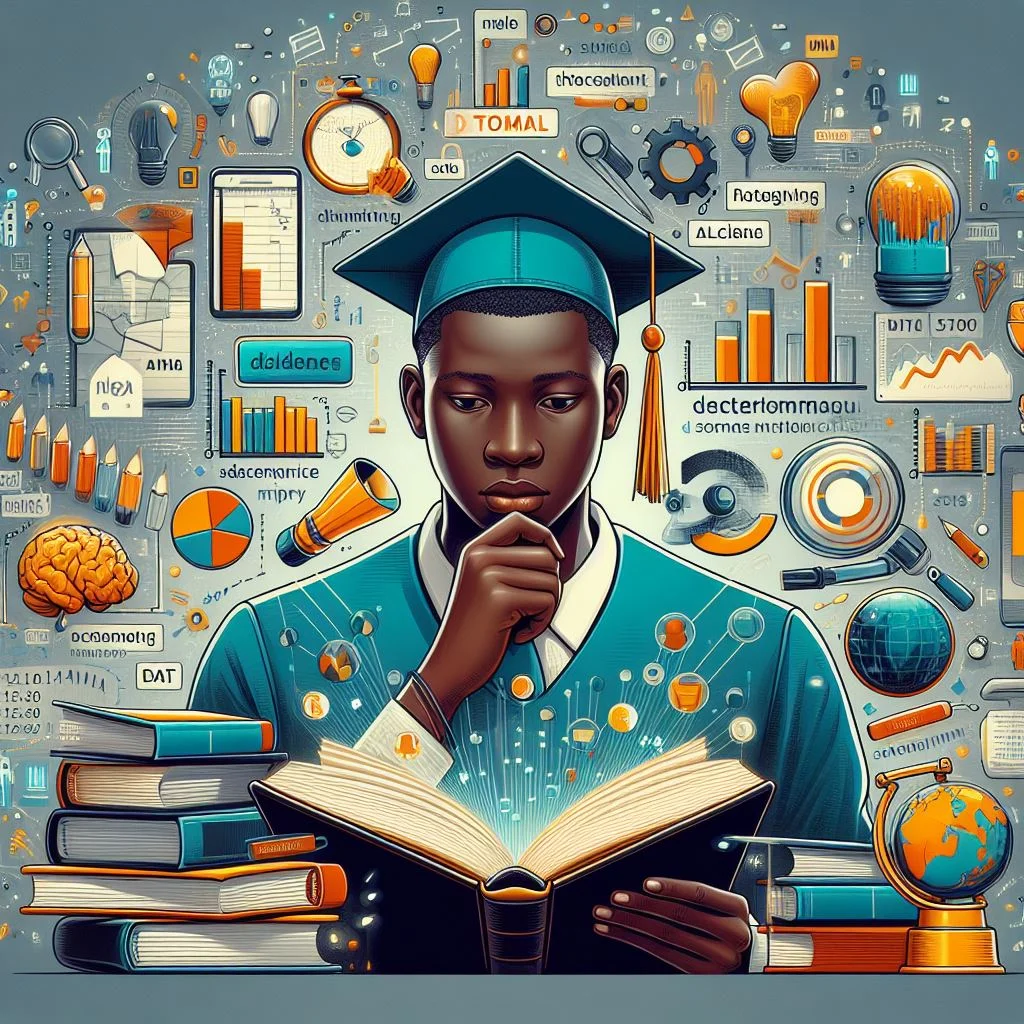
The blog doesn't merely traverse theoretical concepts; it navigates through the application of these theories. Real-world case studies become guiding stars, showcasing how data mining techniques manifest in industries like healthcare, finance, and marketing. Hands-on projects take theory from the abstract into the concrete, providing step-by-step guides for implementation using popular tools and programming languages.
Yet, challenges lie ahead. The blog is not blind to the pitfalls awaiting students in their data mining endeavors. Common stumbling blocks are identified, providing a roadmap for circumvention and refining approaches. Ethical considerations are not overlooked, emphasizing the responsibility that accompanies handling sensitive information. The blog acts as a guardian, offering insights on maintaining ethical standards in the realm of data mining.
Resources for continued learning become essential milestones in this journey. Online courses and tutorials stand as oases of knowledge, providing supplementary guidance for theoretical understanding and practical application. Recommended books serve as compasses, offering in-depth explorations into practical data mining techniques, enriching the understanding of students as they navigate this dynamic landscape.
As the exploration concludes, it becomes evident that the transition from theory to practice is not just a step but a transformative process. The theoretical foundation serves as the launchpad, propelling students into a realm where data mining isn't just a concept but a tangible, impactful skill. The blog, as a comprehensive guide, facilitates this transformation, ensuring that students not only comprehend the theoretical intricacies but also emerge equipped to apply them in real-world scenarios.
In essence, this blog is an odyssey through the world of data mining—an odyssey that transforms theoretical novices into practical maestros. It is a roadmap, a compass, and a guiding light for students embarking on a journey where theory meets practice, and data transforms into valuable insights.
Understanding the Basics
Embarking on the exploration of data mining requires a deep dive into its theoretical foundations. In this segment, we unravel the intricate aspects of data mining, dissecting core concepts, algorithms, and methodologies that constitute the bedrock of this dynamic field. It is here that students will gain a profound understanding of the theoretical underpinnings that govern data mining processes, laying the groundwork for the practical applications that follow. As we navigate through this theoretical landscape, the goal is to equip students with a comprehensive knowledge base, fostering a solid foundation upon which they can build their expertise in the practical realm of data mining.
The significance of a robust theoretical foundation in data mining education cannot be overstated. This section explores the pivotal role that theory plays in shaping the capabilities of students entering the intricate world of data mining. By delving into the "why" behind the theory, we uncover the critical framework that theory provides, serving as a guiding force for practical applications. Students will gain insights into how theoretical knowledge not only informs their understanding of data mining concepts but also empowers them to navigate and solve real-world problems. Understanding the importance of theory becomes the compass guiding students as they traverse the challenging terrain of data mining education, ensuring a more profound and meaningful grasp of the subject matter.
Essential Data Mining Techniques
In the realm of data mining, Classification and Regression Analysis are fundamental techniques that play a crucial role in predicting and categorizing data. Students exploring these methods gain insights into how data can be effectively organized and classified based on specific attributes. The art of regression analysis further equips them to make informed predictions, extracting valuable insights from datasets. This section not only introduces the theoretical underpinnings but also emphasizes the practical significance of classification and regression in unraveling patterns within complex datasets, laying a robust foundation for data mining endeavors.
Navigating the intricate web of data points, Clustering Techniques emerge as a beacon, illuminating the path towards organizing similar elements into cohesive groups. As students delve into the intricacies of clustering, they unravel the art of grouping data points based on inherent similarities, opening doors to a myriad of practical applications. This section goes beyond theoretical discussions, immersing students in practical examples and real-world scenarios where clustering techniques prove instrumental. From market segmentation to image recognition, the applications are diverse, showcasing the versatility of clustering in uncovering patterns that might elude simpler analytical methods. By mastering these techniques, students gain a powerful toolset to categorize, understand, and draw meaningful insights from the vast sea of data they encounter in their data mining pursuits.
The realm of data mining holds many secrets, and Association Rule Mining serves as the key to unlocking hidden patterns and relationships within datasets. This section immerses students in the art of discovering intricate connections, providing insights into the inner workings of this powerful technique. As students delve into real-world applications, they witness firsthand how association rule mining transcends industries, offering valuable perspectives in fields as diverse as retail, healthcare, and finance. By understanding the principles behind association rules, students gain the ability to unravel complex associations within data, paving the way for informed decision-making and strategic planning.
In the dynamic landscape of data, Time Series Analysis emerges as a crucial technique, allowing students to extract meaningful insights from time-ordered data. As they explore the relevance of time series analysis, real-world examples come to life, illustrating its importance in diverse fields such as finance, weather forecasting, and trend analysis. This section goes beyond the theoretical foundations, providing students with practical knowledge to navigate and analyze temporal data. By mastering time series analysis, students acquire a skill set that is essential for predicting trends, understanding patterns, and making informed decisions in time-sensitive scenarios, solidifying their prowess in the realm of data mining.
Practical Applications
Engaging with real-world case studies is a pivotal aspect of mastering data mining techniques. These case studies serve as dynamic examples that seamlessly connect theoretical concepts with actionable insights across various industries. In healthcare, for instance, data mining plays a crucial role in analyzing patient records, identifying patterns, and predicting potential health issues. The practical application of data mining in this field enables healthcare professionals to make informed decisions, personalize treatment plans, and enhance patient care.
Similarly, the financial sector relies heavily on data mining to detect fraudulent activities, assess credit risks, and optimize investment strategies. By examining historical data and patterns, financial institutions can make data-driven decisions that impact not only their operational efficiency but also the overall stability of the financial market. The translation of data mining theory into practical solutions empowers finance professionals to navigate a complex landscape with greater accuracy and foresight.
In the realm of marketing, data mining becomes a powerful tool for understanding consumer behavior, preferences, and trends. Analyzing vast datasets allows marketers to tailor their strategies, optimize advertising campaigns, and enhance customer engagement. Through real-world case studies, students can witness the transformative impact of data mining on marketing outcomes, providing them with insights that extend beyond theoretical knowledge.
Complementing case studies, hands-on projects form a cornerstone of practical education in data mining. These projects offer students the opportunity to apply theoretical concepts in a tangible and interactive manner. By providing step-by-step guides, we aim to facilitate a seamless transition from theory to practice, empowering students to implement data mining techniques using popular tools and programming languages.
Hands-on projects not only reinforce theoretical understanding but also cultivate essential skills for future data scientists. As students navigate through the intricacies of these projects, they develop problem-solving abilities, critical thinking skills, and a deeper appreciation for the real-world impact of data mining. The hands-on approach ensures that students not only grasp the theoretical underpinnings of data mining but also gain the confidence to tackle complex challenges in a professional setting.
The projects are designed to cover a spectrum of data mining techniques, allowing students to explore classification and regression analysis, clustering, association rule mining, and time series analysis. This comprehensive approach ensures that students receive a holistic understanding of data mining and its versatile applications.
In conclusion, the integration of case studies and hands-on projects serves as a bridge between theoretical knowledge and practical application in the field of data mining. These components collectively enrich the learning experience, offering students insights into the real-world implications of their studies and the skills needed to thrive in the ever-evolving landscape of data science. By engaging with case studies and actively participating in hands-on projects, students not only enhance their understanding of data mining but also position themselves for success in a data-driven world.
Overcoming Challenge
Embarking on data mining projects can be fraught with challenges, and students often encounter common pitfalls that hinder the success of their endeavors. From issues related to data quality and preprocessing to the complexities of selecting appropriate algorithms, navigating these obstacles is a crucial aspect of mastering data mining techniques. Understanding the intricacies of feature selection, dealing with imbalanced datasets, and managing computational resources are among the challenges that students must confront. Moreover, the interpretation of results and the potential for overfitting pose additional hurdles. This section will guide students through these common pitfalls, offering insights on how to identify, address, and ultimately overcome these challenges. By developing a nuanced understanding of the stumbling blocks in data mining projects, students can refine their approach, enhance the quality of their analyses, and ensure the success of their data-driven initiatives.
As students delve into the realm of data mining, ethical considerations take center stage in the responsible application of powerful analytical tools. The extraction of valuable insights from data comes with the responsibility to handle information ethically, especially when dealing with sensitive data. This section explores the ethical implications of data mining practices, emphasizing the importance of privacy, consent, and transparency. Students will gain insights into the ethical dilemmas associated with data collection, storage, and usage. Additionally, the discussion delves into the societal impact of data mining, addressing issues of bias, discrimination, and the potential misuse of information. By engaging with best practices and ethical guidelines, students will be equipped to navigate the complex landscape of data mining responsibly, ensuring that their analyses contribute positively to both academic and real-world contexts.
Resources for Continued Learning
Discover a wealth of online courses and tutorials designed to complement theoretical learning with practical applications in the dynamic field of data mining. These resources serve as invaluable tools for students looking to hone their skills, providing a bridge between theory and hands-on implementation. From introductory courses to advanced tutorials, students can access a variety of learning materials tailored to different proficiency levels. Engage with interactive modules, video lectures, and practical exercises, empowering yourself to navigate the complexities of data mining with confidence. Stay abreast of the latest developments in the digital landscape through these dynamic and flexible online resources.
Embark on a knowledge exploration journey with recommended books offering an in-depth look into practical data mining techniques. Carefully curated, these resources serve as valuable references, providing comprehensive insights and practical guidance. Authored by experts in the field, these books cover a wide array of topics, from foundational concepts to advanced methodologies. Delve into case studies, real-world applications, and practical examples that illuminate the intricacies of data mining. These recommended books not only facilitate a deep understanding of the subject matter but also empower readers to apply their knowledge in diverse contexts. Whether you are a beginner seeking a solid foundation or an experienced practitioner looking to refine your skills, these books offer a wealth of information to support your journey in mastering practical data mining techniques.
Conclusion
In our journey through the realm of data mining, it becomes increasingly apparent that the transition from theory to practice is not just a bridge but a crucial crossroads for students aiming to master data mining techniques. Understanding the basics is akin to laying the foundation stones, providing stability and structure to the edifice of knowledge. It is the theoretical groundwork that equips students with the vocabulary and conceptual frameworks necessary to comprehend the intricacies of data mining.
However, the theoretical framework alone is akin to having a map without embarking on the journey. The landscape of data mining is rich and varied, comprising diverse methodologies and algorithms. To truly master this field, students must go beyond rote understanding and engage in the exploration of various methods. This involves delving into the mechanics of classification and regression analysis, understanding how data points can be categorized and predicted. It extends to the world of clustering techniques, where the grouping of similar data points reveals patterns and associations not immediately apparent. Association rule mining takes students further, unveiling hidden relationships within datasets, while time series analysis adds a temporal dimension, extracting insights from time-ordered data.
Yet, the true revelation occurs when these theoretical constructs metamorphose into practical applications. The synergy between theoretical knowledge and hands-on experience is the alchemy that unlocks the full potential of data mining. It is in the application of these techniques to real-world scenarios that students truly grasp the power and significance of data mining. Case studies become windows into industries where data mining is not just a theoretical construct but a practical necessity, be it in healthcare, finance, or marketing.
To solidify this understanding, students must not merely observe but actively participate in the process. Hands-on projects offer a crucible where theoretical knowledge is tested and refined in the fires of practical application. These projects, often involving popular tools and programming languages, serve as the proving ground for students to hone their skills, transforming theoretical understanding into actionable insights.
However, this journey is not without its challenges. Practical application brings its own set of hurdles, from data cleaning and preprocessing to the selection of appropriate algorithms. Students must navigate these challenges to truly harness the power of data mining. Moreover, ethical considerations come to the forefront, as the handling of sensitive information demands a responsible approach. These challenges, though formidable, are essential crucibles for the forging of skilled data miners.
As we conclude this exploration, it is evident that the symbiosis between theory and practice is not just desirable but essential. The theoretical framework provides the map, guiding students through the vast landscape of data mining. Practical application, on the other hand, is the compass that directs their steps, ensuring they don't merely traverse the theoretical terrain but also make meaningful contributions to the real-world landscape of data science. It is this fusion that propels students from the realm of understanding into the domain of expertise, where data mining is not just a theoretical concept but a potent tool in their hands, ready to unravel the complexities of the real world.