Predictive Modeling in Assignment Help: Harnessing the Future with Advanced Data Mining Techniques
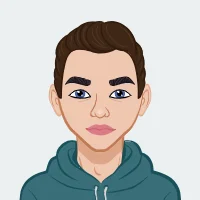
In the ever-evolving landscape of education, technological advancements continue to play a pivotal role. Predictive modeling, coupled with advanced data mining techniques, has emerged as a powerful tool in the realm of assignment help. This blog will delve into the fascinating world of predictive modeling, exploring how it is revolutionizing the way students approach their academic challenges, offering assistance with your data mining homework and ensuring proficiency in this essential aspect of modern education.
In the journey to comprehend the role of predictive modeling in education, it is imperative to delve into its essence. Predictive modeling involves the utilization of statistical algorithms and advanced machine learning techniques to scrutinize historical data meticulously. This analytical process transcends traditional methodologies, paving the way for predicting future outcomes. Within the realm of assignment help, predictive modeling becomes a guiding light, offering educators insights into student performance trends and enabling them to anticipate challenges effectively.
In tandem with predictive modeling, data mining techniques emerge as the driving force that propels the educational landscape forward. Data mining, the art of extracting patterns and insights from vast datasets, becomes a beacon of illumination in the world of assignment help. By sifting through student records, feedback mechanisms, and performance metrics, educators can unlock a treasure trove of information. This data-driven approach not only enhances our understanding of student behaviors and learning patterns but also serves as the catalyst for informed decision-making.
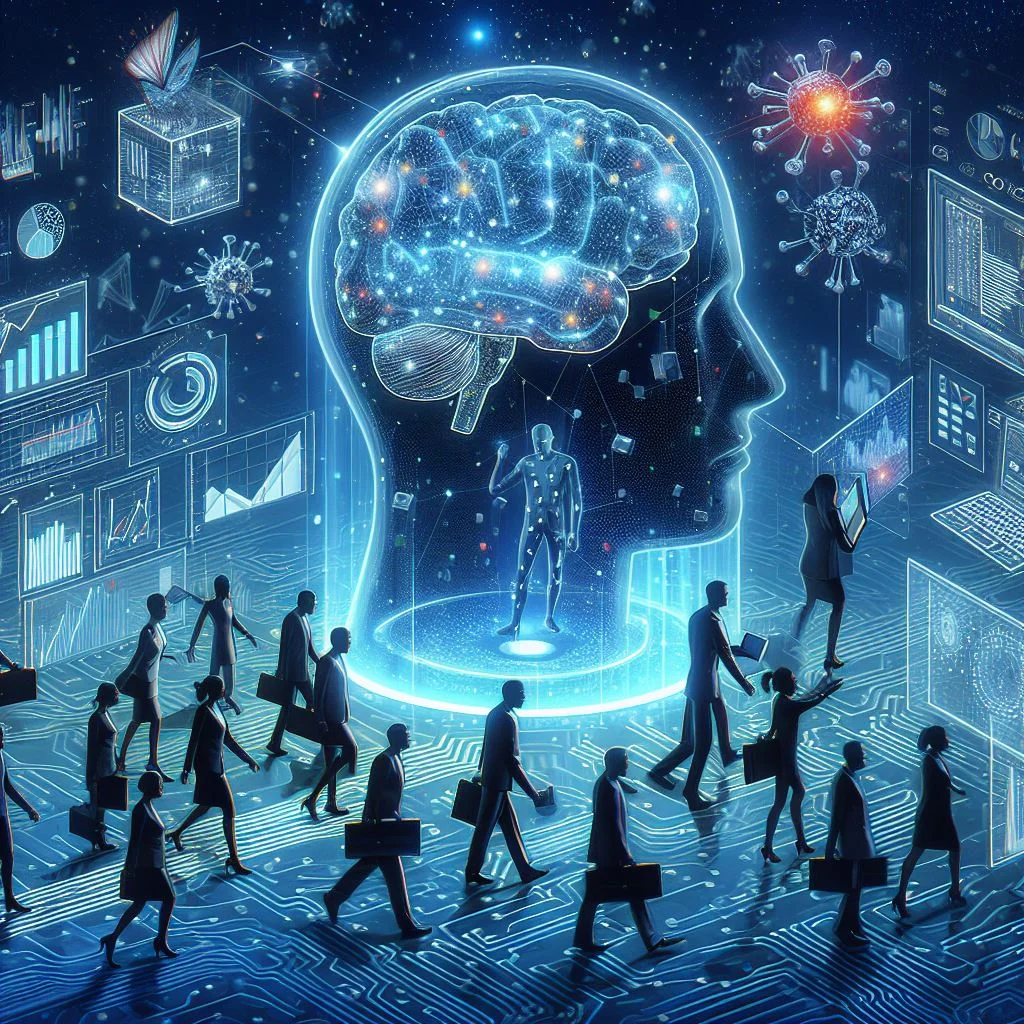
At the heart of predictive modeling's impact lies the ability to tailor learning experiences to the unique needs of individual students. By harnessing the power of predictive insights, educators can craft assignments that resonate with each student's distinctive learning style. This personalized approach fosters a more engaging and effective learning environment, where assignments transcend the one-size-fits-all model, catering to the diverse needs of a dynamic student body. Precision in grading and feedback processes stands as a testament to the transformative potential of predictive modeling in assignment help. The incorporation of historical data into the evaluation process enables a more accurate and efficient grading system. By identifying common errors and analyzing successful assignment patterns, educators can provide targeted feedback, streamlining the learning journey for students while optimizing time and resources.
In drawing the curtains on this exploration of predictive modeling in assignment help, the profound impact on education is evident. This amalgamation of predictive modeling and advanced data mining techniques paints a future where personalized learning becomes the norm, academic challenges are addressed proactively, and the ethical dimensions of technology are carefully navigated. As we continue to embrace these technological advancements, the journey toward an educational landscape enriched by predictive insights promises a future where students and educators alike can thrive in an environment that is both innovative and ethically responsible.
Understanding Predictive Modeling
Predictive modeling, at the nexus of statistical algorithms and machine learning, stands as a transformative force in the realm of assignment help. This methodology involves analyzing historical data to make informed predictions about future outcomes. In the context of assignment assistance, predictive modeling becomes a potent tool, guiding educators in anticipating challenges and proactively steering students toward academic success. Complementing this approach are data mining techniques, serving as navigators delving deep into vast datasets to unearth patterns and insights. In the context of assignment help, data mining becomes a powerful lens through which educators can extract valuable information from student records, feedback, and performance metrics. It's the conduit through which a richer understanding of student behavior and learning patterns is achieved. Personalized learning, a cornerstone of modern education, finds new dimensions with the integration of predictive modeling. By discerning patterns in student behavior and preferences, educators can tailor assignments to meet the unique needs of each learner. At the heart of academic success lies accurate assessment and constructive feedback. Predictive modeling, by virtue of its ability to analyze historical data, becomes a game-changer in the realm of grading and feedback. It ensures that students receive targeted feedback, elevating the grading process. Predictive modeling doesn’t just predict; it empowers educators to intervene proactively, addressing potential academic challenges before they escalate. By analyzing factors such as attendance, participation, and past performance, educators can offer timely support and resources. As technology evolves, so does the landscape of predictive modeling. The integration of artificial intelligence (AI) takes predictive modeling to new heights. The adaptability and evolution inherent in AI algorithms promise even more accurate predictions and personalized recommendations, redefining the future of assignment help. In the pursuit of educational advancements through predictive modeling, ethical considerations emerge as a crucial facet. This section navigates the delicate balance between leveraging predictive insights for educational improvements and safeguarding individual privacy. From implementing robust security measures to ensuring transparent data usage, it delves into the ethical dimensions surrounding the responsible application of predictive modeling in the educational landscape. In the grand tapestry of education, predictive modeling emerges not only as a tool for anticipation but as a guiding force for positive transformation. As educators and institutions harness the power of predictive modeling and data mining techniques, the landscape of assignment help is redefined. This concluding section underscores the imperative to navigate ethical considerations responsibly, ensuring that the future of education is one where technology and human values coexist harmoniously, empowering students to thrive in an ever-evolving educational landscape..
The Role of Data Mining Techniques
In the educational landscape, the process of data mining emerges as a potent force, capable of unraveling patterns and insights from expansive datasets. This methodology, akin to an ardent explorer navigating uncharted territories, delves into the depths of information to uncover valuable gems. In the specific context of assignment help, data mining techniques assume a pivotal role, serving as an adept compass for educators. These techniques enable the extraction of invaluable information from diverse sources such as student records, feedback loops, and performance metrics.
In the intricate tapestry of education, this data-driven approach becomes a beacon, illuminating the path towards a deeper understanding of student behavior and learning patterns. No longer reliant solely on anecdotal evidence or traditional assessment methods, educators can harness the power of data mining to gain nuanced insights into the intricacies of student engagement, comprehension, and progress. It transforms the educational landscape from one of conjecture to one of precision, where decisions are informed by empirical evidence extracted from the vast reservoir of available data.
This section navigates the profound impact of data mining in assignment help, highlighting its role as a catalyst for facilitating a more profound connection with student dynamics. It sheds light on how data-driven insights empower educators to tailor their approaches, creating a more responsive and personalized learning environment that goes beyond conventional methodologies. As we journey through the realms of data mining, it becomes evident that the real treasure lies not just in the data itself but in the transformative potential it holds for shaping the future of education.
Enhancing Personalized Learning
In the dynamic realm of education, predictive modeling emerges as a game-changer, unlocking the ability to tailor learning experiences to the unique needs of individual students. This transformative capability stands as one of the key benefits of predictive modeling in the context of assignment help. It represents a departure from the one-size-fits-all approach to education, ushering in an era where assignments are meticulously crafted to align with each student's distinct learning style, preferences, and capabilities.
The crux of this personalized approach lies in the thorough analysis of past performance and preferences. Predictive modeling, equipped with the ability to sift through historical data, becomes a guiding compass for educators. By understanding how each student has performed in the past, educators can glean insights into their strengths, weaknesses, and areas for improvement. This granular understanding allows for the creation of assignments that are not only academically challenging but also tailored to resonate with each student's cognitive processes.
The process begins with an in-depth examination of past assignments, assessments, and learning patterns. Predictive modeling algorithms can identify trends and correlations, providing educators with a comprehensive view of each student's academic journey. This wealth of information empowers educators to design assignments that cater to diverse learning styles, ensuring that the material is not only comprehensible but also optimally engaging.
Moreover, predictive modeling takes into account individual preferences, be it in terms of subject matter, format, or even mode of assessment. This level of personalization extends beyond traditional pedagogical boundaries, recognizing and adapting to the unique nuances that make each student an individual learner. It fosters a learning environment where students feel seen and understood, enhancing their motivation and commitment to the educational process.
The result is a more engaging and effective learning environment, where assignments cease to be mere tasks and transform into personalized learning experiences. Students are no longer passive recipients but active participants in their education, embarking on assignments that not only challenge them but also align with their intrinsic motivations. This tailored approach contributes to a positive feedback loop, where students find joy and satisfaction in their academic pursuits, further fueling their desire to excel.
In conclusion, the ability to tailor assignments to individual needs represents a revolution in assignment help facilitated by predictive modeling. It transcends the limitations of traditional education, paving the way for a more inclusive, adaptive, and student-centric approach. As educators harness the power of predictive modeling, they become architects of personalized learning experiences, shaping a future where each student's unique journey is not just acknowledged but celebrated in the rich tapestry of education.
Improving Assignment Accuracy
In the intricate world of education, predictive modeling emerges as a formidable ally, wielding the power to revolutionize the accuracy of grading and feedback processes. This transformative capability stands as a testament to the evolving landscape of assessment methodologies, where the meticulous analysis of historical data takes center stage. Predictive modeling becomes the discerning lens through which educators can achieve a level of precision previously unattainable, creating a ripple effect that not only saves valuable time but also enhances the quality of feedback for students' continual improvement.
At the core of this precision lies the ability of predictive modeling to delve into the historical data on successful assignments and common errors. This analytical prowess allows the system to identify patterns, trends, and nuances that might elude a traditional grading approach. By learning from the successes and pitfalls of the past, educators can fine-tune their assessment criteria, ensuring a more accurate evaluation of each student's work.
The practical implications of this precision are multifaceted. Firstly, it significantly streamlines the grading process, alleviating the time burden on educators. Predictive modeling automates the identification of common errors and benchmarks for success, enabling educators to focus their attention on the nuanced aspects of each assignment rather than spending excessive time on routine assessments.
However, the true magic unfolds in the realm of feedback. Predictive modeling not only facilitates accurate grading but also ensures that feedback is targeted and tailored to address specific areas of improvement. Instead of generic comments, students receive insights that pinpoint the precise aspects where they excelled and areas that demand attention. This individualized feedback becomes a roadmap for students, guiding them on their educational journey with clarity and purpose.
Moreover, the continuous feedback loop established by predictive modeling cultivates a culture of improvement. Students, armed with specific insights into their strengths and weaknesses, are empowered to refine their approach to learning. This iterative process fosters a sense of ownership and accountability, as students actively engage in the feedback provided, driving their progress forward.
In conclusion, the precision in grading and feedback facilitated by predictive modeling heralds a new era in educational assessment. It transcends the limitations of traditional grading methods, offering a nuanced and individualized approach that benefits both educators and students. As predictive modeling becomes an integral part of the educational toolkit, it not only optimizes time and resources but also contributes to the holistic development of students, shaping a future where assessments are not just evaluative measures but valuable learning opportunities.
Overcoming Academic Challenges
In the ever-evolving landscape of education, predictive modeling emerges as a powerful ally, reshaping the paradigm of student support through early intervention. This transformative capability becomes a beacon for educators, enabling them to identify students at risk of falling behind before academic challenges escalate. By delving into a myriad of factors such as attendance, participation, and previous performance, predictive modeling becomes a proactive tool, allowing educators to extend timely support and resources, fostering an environment where no student is left behind.
At the heart of this proactive approach lies the nuanced analysis enabled by predictive modeling. By scrutinizing various facets of a student's academic journey, educators can uncover patterns and trends that might indicate potential challenges. Whether it's a decline in attendance, a drop in participation, or a deviation from previous performance metrics, predictive modeling becomes the attentive guardian, signaling when a student might be veering off the path to academic success.
The early identification facilitated by predictive modeling serves as a crucial intervention point. It allows educators to reach out to at-risk students, offering targeted support tailored to their specific needs. This intervention can take various forms, including additional tutoring, personalized study plans, or counseling sessions, depending on the nature of the challenges detected. The goal is not just to remediate academic struggles but to address the root causes comprehensively.
Beyond academic support, early intervention contributes to the overall well-being of students. It acknowledges that academic challenges often have multifaceted origins, including personal or socio-economic factors. By recognizing these complexities through predictive modeling, educators can collaborate with support services to offer a holistic support system that extends beyond the classroom.
Moreover, the early intervention mindset instilled by predictive modeling is a testament to the proactive and preventative approach in education. Instead of waiting for issues to escalate, educators become partners in a student's academic journey, working collaboratively to navigate challenges before they become insurmountable. This not only promotes a positive and supportive learning environment but also cultivates a sense of trust between educators and students.
In conclusion, early intervention for at-risk students facilitated by predictive modeling stands as a cornerstone in modern education. It transcends traditional reactive approaches, ushering in an era where challenges are met with proactive solutions. As predictive modeling becomes an integral part of the educational toolkit, it not only identifies at-risk students but also transforms the educational landscape into one where timely support and resources pave the way for every student to overcome challenges and thrive academically.
The Ethical Dimensions
In the dynamic intersection of education and technology, predictive modeling emerges as a transformative force, providing invaluable insights into student performance. Yet, as this powerful tool unlocks the potential for educational improvements, a critical ethical consideration looms large: the delicate balance between leveraging predictive insights and safeguarding individual privacy.
The benefits of predictive modeling are undeniable, offering educators the ability to anticipate challenges, personalize learning experiences, and provide timely interventions. However, the ethical responsibility to protect students' privacy remains paramount. Striking this delicate balance requires a meticulous approach, with an unwavering commitment to respecting the rights and confidentiality of individuals.
One of the foundational principles of responsible predictive modeling is the implementation of robust security measures. This entails safeguarding the data collected, ensuring it remains protected from unauthorized access or misuse. Encryption protocols, secure servers, and stringent access controls become the fortifications that shield sensitive student information from potential breaches. The assurance of a secure data environment is not just a technological necessity but a moral imperative in the age of digital education.
Transparency becomes another cornerstone of ethical predictive modeling. Educators, administrators, and institutions must communicate openly about the collection, usage, and storage of student data. Transparent practices ensure that students and their families are aware of how their information is being utilized, fostering trust and accountability. This transparency extends to explaining the purpose of predictive modeling, the types of data involved, and the measures in place to protect privacy.
An essential aspect of ethical predictive modeling is the concept of data minimization – the practice of collecting only the data necessary for the intended purpose. By limiting the scope of data collection to what is directly relevant for educational insights, the risk of privacy infringement diminishes. This principle underscores the ethical obligation to prioritize the educational value derived from predictive modeling while minimizing any potential intrusion into students' private lives.
Moreover, informed consent becomes a linchpin in ethical data usage. Institutions must obtain explicit consent from students or their legal guardians before implementing predictive modeling initiatives. This consent should be comprehensive, clearly articulating the nature of data collection, the purposes it serves, and the protective measures in place. In doing so, educators uphold the ethical principle of autonomy, allowing individuals to make informed decisions about the use of their data.
As we navigate the ethical horizon of predictive modeling, ongoing scrutiny and evaluation of practices become imperative. Regular audits, assessments, and feedback loops ensure that ethical standards are not only established but consistently upheld. Institutions must remain agile in adapting their practices to evolving ethical norms, technological advancements, and legal frameworks governing data protection.
In conclusion, while predictive modeling holds immense potential for educational enhancements, its ethical implementation is non-negotiable. Balancing predictive insights and privacy requires a conscientious commitment to robust security measures, transparent communication, data minimization, informed consent, and ongoing scrutiny. As educators harness the power of predictive modeling, they must not only elevate academic outcomes but also champion the ethical principles that safeguard the privacy and dignity of every student in the digital era of education.
Conclusion
In the ever-evolving landscape of education, predictive modeling in assignment help stands as a beacon of transformative change. This innovative approach, fueled by the power of advanced data mining techniques, marks a paradigm shift in how we perceive and engage with the educational process. As educators harness these technologies, a new era of personalized learning experiences and improved academic outcomes unfolds before us.
The integration of predictive modeling empowers educators to unlock insights that were once elusive. By delving into the intricate web of data, they can discern patterns, anticipate challenges, and tailor learning experiences to the unique needs of each student. This shift from a standardized approach to a personalized one not only enhances student engagement but also fosters a deeper understanding of individual learning styles, preferences, and potential hurdles.
The marriage of predictive modeling and advanced data mining techniques transcends traditional boundaries, providing educators with a dynamic toolkit for shaping the educational journey. It brings forth a future where assignments are not generic tasks but finely crafted experiences, assessments are not merely evaluative but insightful, and interventions are not reactive but proactive.
However, as we navigate this frontier of technological advancement, it is crucial to tread carefully through the ethical considerations that accompany these innovations. The responsible use of predictive modeling requires a steadfast commitment to safeguarding individual privacy. As we embrace the benefits of these technological advancements, we must concurrently navigate the ethical landscape, ensuring that education technology becomes a force for good without compromising the fundamental rights and privacy of each student.
In this transformative journey, educators become architects of a future where technology augments, rather than replaces, the human touch in education. Predictive modeling becomes a collaborator, a guide that enriches the educational experience and empowers both educators and students. Through a thoughtful and ethical application of these technologies, we pave the way for an educational landscape where every student can thrive, learn, and excel, confident in the knowledge that their privacy and dignity are held sacrosanct. As we embark on this educational odyssey, the fusion of predictive modeling and ethical considerations becomes the compass that guides us toward a future where technology and humanity harmoniously coexist for the betterment of education.
In the relentless pursuit of educational excellence, predictive modeling, accompanied by advanced data mining techniques, propels us into a future where the boundaries of learning are pushed ever further. The profound insights derived from predictive modeling not only revolutionize assignments and assessments but also pave the way for a more adaptive and responsive educational ecosystem.
As we stand at the nexus of technology and education, the potential for growth is boundless. The integration of predictive modeling fosters an environment where every student is seen, understood, and supported on an individualized journey. The traditional constraints of standardized teaching methods dissolve, making room for a diverse tapestry of learning experiences tailored to each student's unique strengths and challenges.
However, with great technological strides comes an equal responsibility to navigate the ethical considerations diligently. We must ensure that the promise of enhanced education is not achieved at the expense of individual privacy. Striking this delicate balance requires a continuous commitment to transparency, security, and ethical practices, safeguarding the rights and dignity of each learner.